MEDIA ANALYTICS
PROJECTS
PEOPLE
PUBLICATIONS
PATENTS
Hierarchical Metric Learning & Matching for 2D & 3D Geometric Correspondences
While a metric loss applied to the deepest layer of a CNN is expected to yield ideal features, the growing receptive field and striding effects cause shallower features to be better at high-precision matching. We leverage this insight, along with hierarchical supervision, to learn more effective descriptors for geometric matching. We evaluate for 2D and 3D geometric matching as well as optical flow, demonstrating state-of-the-art results and generalization across multiple datasets.
Collaborators: Mohammed E. Fathy, Quoc-Huy Tran, M. Zeeshan Zia, Paul Vernaza, Manmohan Chandraker
Project Site
Our hierarchical metric learning retains the best properties of various levels of abstraction in CNN feature representations. For geometric matching, we combine the robustness of deep layers that imbibe greater invariance, with the localization sensitivity of shallow layers. This allows learning better features, as well as a better correspondence search strategy that progressively exploits features from higher recall (robustness) to higher precision (spatial discrimination).
Hierarchical Metric Learning and Matching for 2D and 3D Geometric Correspondences Paper
[Download Paper] [The CVF PDF] [Supplemental Paper] [Bibtex]
Abstract
2D Correspondence Results
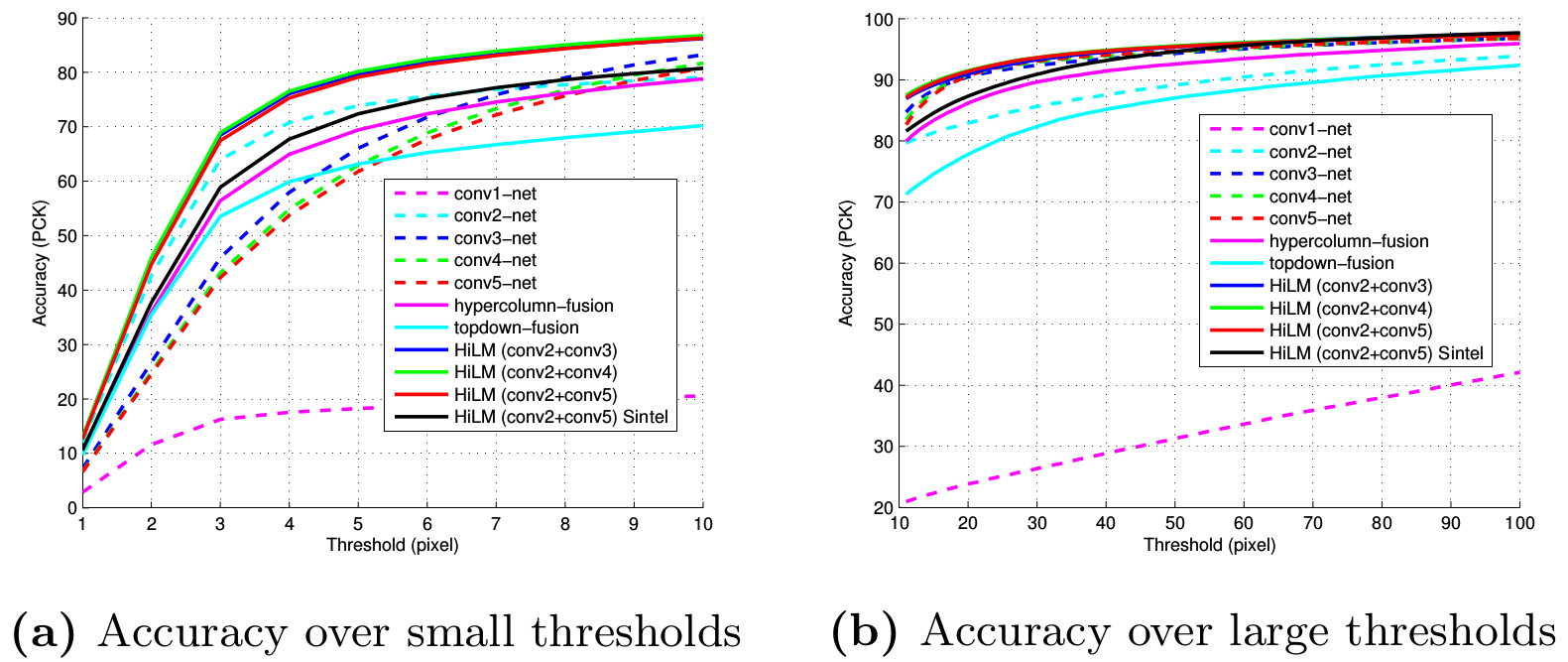
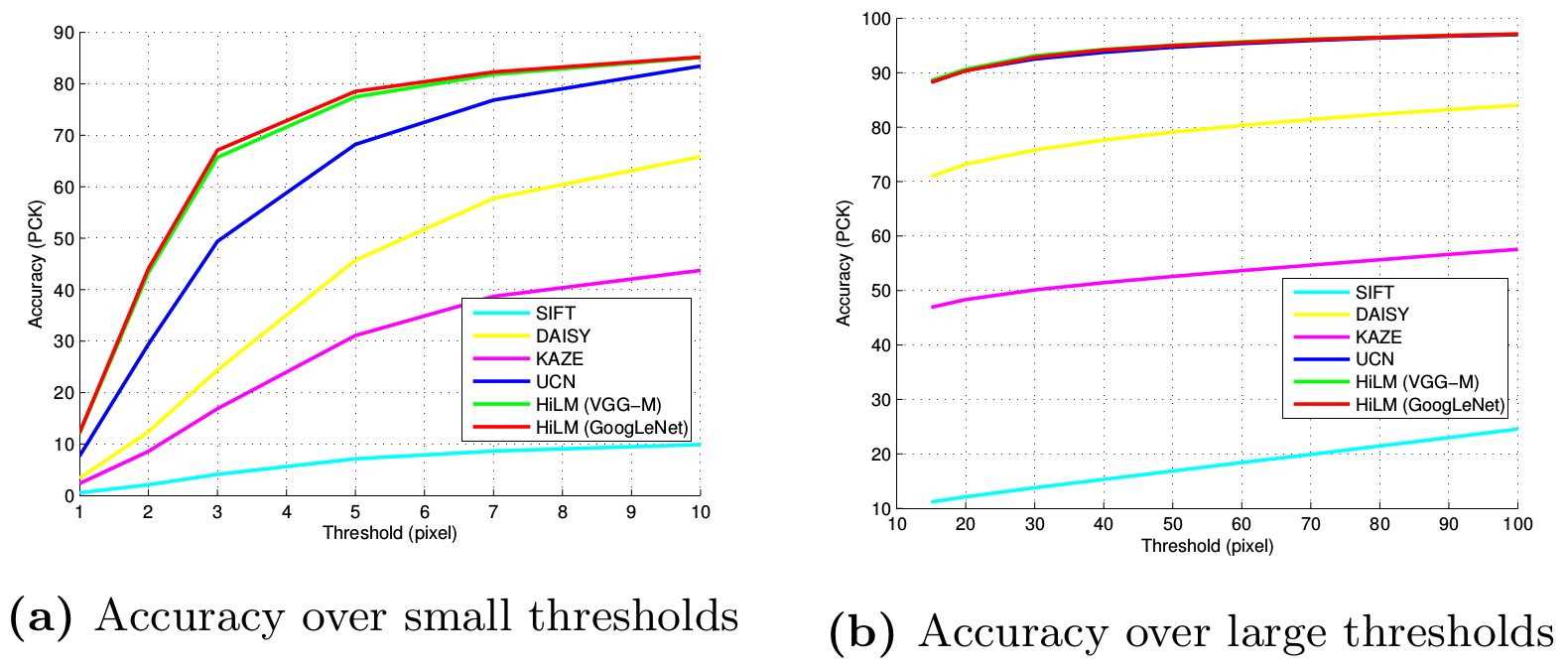
Optical Flow Results
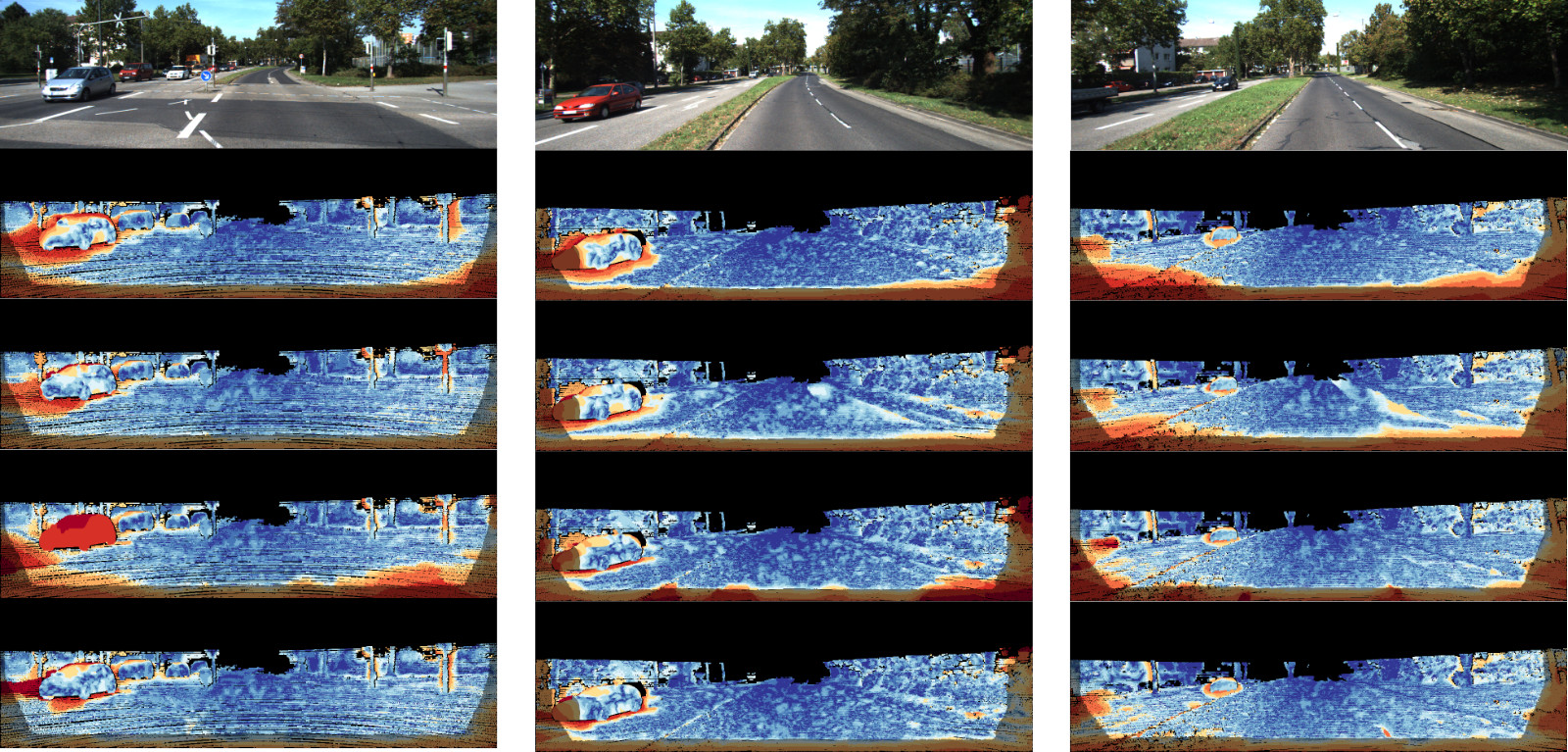
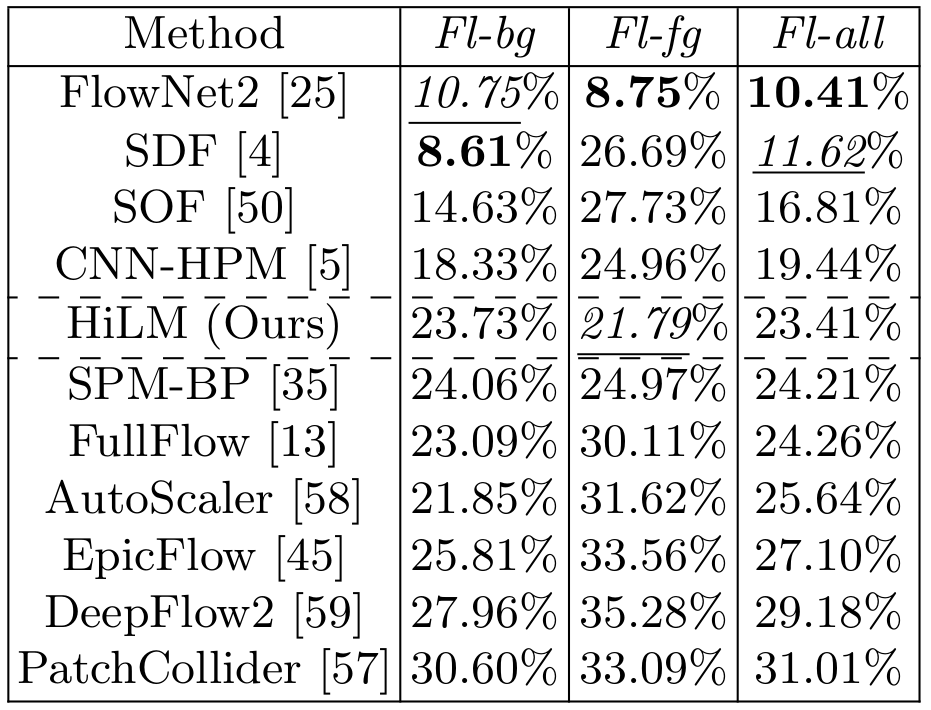
3D Correspondence Results
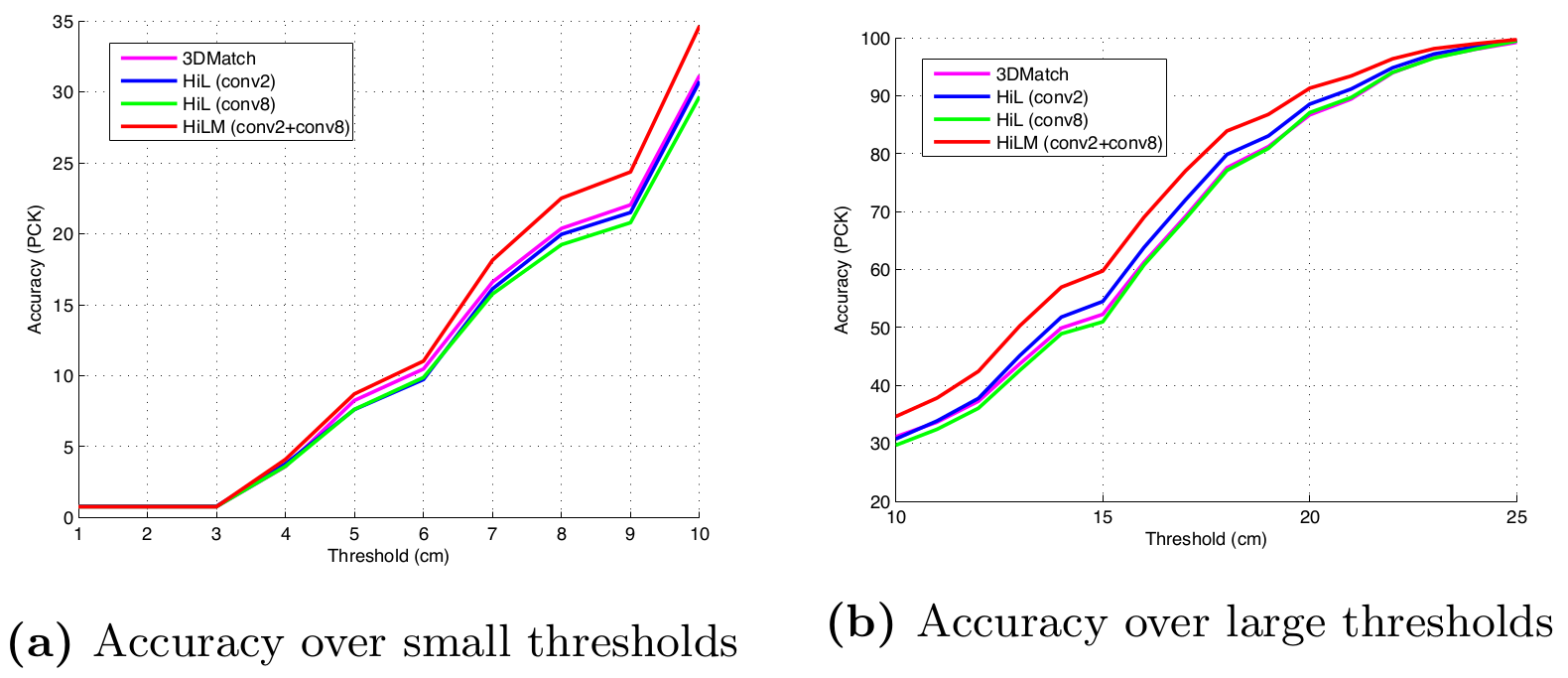