Cheap Supervision refers to a training approach where the model is trained with minimal or inexpensive labeling efforts. Instead of relying on a large amount of precisely labeled data, cheap supervision methods often leverage heuristics, weak labels, or other cost-effective annotation strategies. The goal is to reduce the manual labeling cost while still achieving acceptable performance.
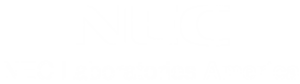
Contact Us
Princeton Office
4 Independence Way, Suite 200
Princeton, NJ 08540
+1 609-520-1555
San Jose Office
2033 Gateway Place, Suite 200
San Jose, CA 95110
+1 408-863-6007
About Us
NEC Laboratories America, Inc. (NEC Labs) is the US-based center for NEC Corporation’s global network of corporate research laboratories. Our diverse research groups collaborate with industry, academia and governments to provide disruptive solutions to complex problems. A leader in the integration of IT and network technologies with more than 100 years of expertise, NEC provides a combination of products and solutions that cross-utilize the company’s experience and global resources to meet the complex and ever-changing needs of its customers.
Our Pages
Read Our Blog Posts
- NEC Labs America Team Attending CVPR 2024 in Seattle
- Multi-Agent Simulator for Carbon Neutrality: The Technology the World Has Been Waiting For
- NEC Labs America at OFC 2024 San Diego from March 24 – 28
- NEC Laboratories Advances Material Design with AI-based MateriAI Platform
- Apply for a Summer 2024 Internship
- Unearthing Nature’s Orchestra – How Fiber Optic Cables Can Hear Cicada Secrets
- NEC Labs America Team Heading to NeurIPS23 in New Orleans
- Sarper Ozharar Receives Award from Koç University
- Meet the NEC Labs America Intern Helping to Make Autonomous Vehicles Safer and More Secure
- AI/Fiber-Optic Combo Poised To Improve Telecommunications
- Industrial Labs to Drive Disruptive Innovation for the Fourth Industrial Revolution
- A New Hope: AI Research is Conquering Today’s Computer Vision Plateau
- NEC Labs America’s Time Series Data Research Drives Space Systems Innovation
- Next-Generation Computing Finally Sees Light
- AI/Fiber-Optic Combo Poised To Improve Telecommunications
- Using AI To Safely Put The First Woman On The Moon
- Our AI Research Contributing to NASA’s Artemis Space Program
- NEC provides AI-based traffic monitoring system with fiber-optic sensing technology for NEXCO CENTRAL
- Beyond Communication: Telecom Fiber Networks for Rain Detection and Classification