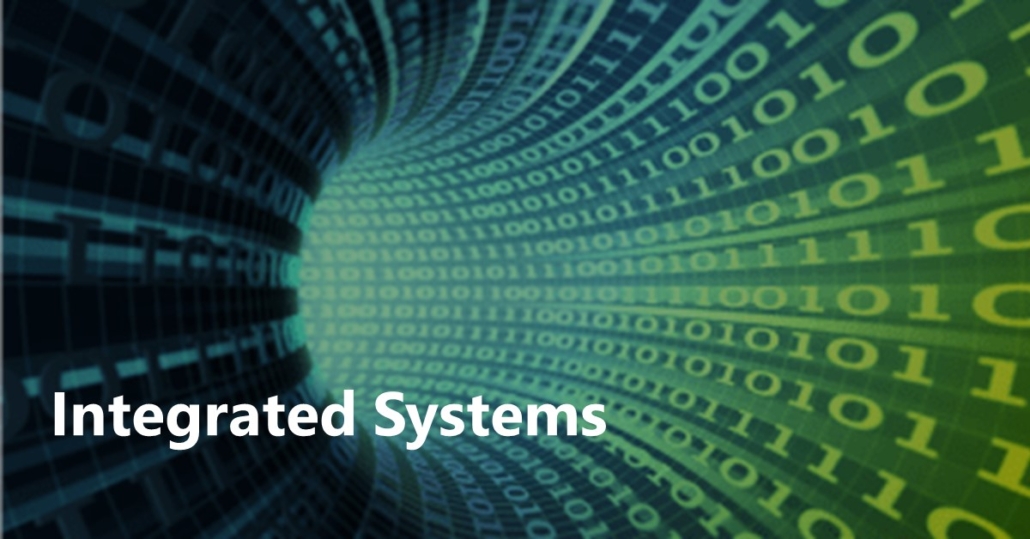
Our Integrated Systems department innovates, designs, and prototypes high-performance intelligent distributed systems, applications, and services on complex, large-scale communication networks like 5G and beyond. We develop next-generation wireless technologies for sensing the world, localizing critical assets, and improving the capacity, coverage, and scalability of communication networks like 5G and beyond.
New application needs have always sparked human innovation. A decade ago, cloud computing enabled high-value enterprise services with a global reach and scale but with several minutes or seconds of delay. Large-scale services like enterprise resource planning (ERP) were a corner-case scenario, often designed as one-off systems. Today, applications like social networks, automated trading, and video streaming have made large-scale services the norm rather than the exception. In the future, advances in 5G networks and an explosion in smart devices, microservices, databases, networking, and computing tiers will make services so complex that humans cannot tune or manage them.
The sheer scale, dynamic nature, and concurrency in services on 5G slices will require them to be intelligent and autonomic. They will need to continuously self-assess, learn, and automatically adjust for resource needs, data quality, and service reliability. The need for increased efficiency and reduced latency between measurement and action drives our design of real-time distributed systems for feature extraction, computation, and machine learning on multimodal streaming data. We are conducting extensive research on creating end-to-end solutions using multimodal sensing technologies in the retail, public safety, and transportation domains.
Our 5G cellular network research encompasses the development of technologies on the Radio Access Network (RAN), the mobile edge, and the 5G LAN. Within the RAN, we are developing technologies that optimize massive MIMO/MU-MIMO deployments and millimeter-wave access (e.g., transmission at 28 GHz to nomadic/mobile users). At the mobile edge (MEC), we focus on virtualization, scalability, and cloud deployment of appropriate services. Our 5G LAN research extends the benefits of 5G slicing technology to enterprise LANs to position the enterprise as the new MEC.
Read our news and publications from our world-class team of researchers from our Integrated Systems department.
The transformer structure employed in large language models (LLMs), as a specialized category of deep neural networks (DNNs) featuring attention mechanisms, stands out for their ability to identify and highlight the most relevant aspects of input data. Such a capability is particularly beneficial in addressing a variety of communication challenges, notably in the realm of semantic communication where proper encoding of the relevant data is critical especially in systems with limited bandwidth. In this work, we employ vision transformers specifically for the purpose of compression and compact representation of the input image, with the goal of preserving semantic information throughout the transmission process. Through the use of the attention mechanism inherent in transformers, we create an attention mask. This mask effectively prioritizes critical segments of images for transmission, ensuring that the reconstruction phase focuses on key objects highlighted by the mask. Our methodology significantly improves the quality of semantic communication and optimizes bandwidth usage by encoding different parts of the data in accordance with their semantic information content, thus enhancing overall efficiency. We evaluate the effectiveness of our proposed framework using the TinyImageNet dataset, focusing on both reconstruction quality and accuracy. Our evaluation results demonstrate that our framework successfully preserves semantic information, even when only a fraction of the encoded data is transmitted, according to the intended compression rates.
https://neclabs.wpengine.com/wp-content/uploads/2022/08/NEC-Labs-Blue-Logo-Square-300x267.jpg
0
0
NEC Labs America
https://neclabs.wpengine.com/wp-content/uploads/2022/08/NEC-Labs-Blue-Logo-Square-300x267.jpg
NEC Labs America2024-10-27 00:00:002025-04-24 10:11:32Transformer-Aided Semantic CommunicationsRetrieval-augmented generation (RAG) systems combine the strengths of language generation and information retrieval to power many real-world applications like chatbots. Use of RAG for understanding of videos is appealing but there are two critical limitations. One-time, upfront conversion of all content in large corpus of videos into text descriptions entails high processing times. Also, not all information in the rich video data is typically captured in the text descriptions. Since user queries are not known apriori, developing a system for video to text conversion and interactive querying of video data is challenging.To address these limitations, we propose an incremental RAG system called iRAG, which augments RAG with a novel incremental workflow to enable interactive querying of a large corpus of videos. Unlike traditional RAG, iRAG quickly indexes large repositories of videos, and in the incremental workflow, it uses the index to opportunistically extract more details from select portions of the videos to retrieve context relevant to an interactive user query. Such an incremental workflow avoids long video to text conversion times, and overcomes information loss issues due to conversion of video to text, by doing on-demand query-specific extraction of details in video data. This ensures high quality of responses to interactive user queries that are often not known apriori. To the best of our knowledge, iRAG is the first system to augment RAG with an incremental workflow to support efficient interactive querying of a large corpus of videos. Experimental results on real-world datasets demonstrate 23x to 25x faster video to text ingestion, while ensuring that latency and quality of responses to interactive user queries is comparable to responses from a traditional RAG where all video data is converted to text upfront before any user querying.
https://neclabs.wpengine.com/wp-content/uploads/2022/08/NEC-Labs-Blue-Logo-Square-300x267.jpg
0
0
NEC Labs America
https://neclabs.wpengine.com/wp-content/uploads/2022/08/NEC-Labs-Blue-Logo-Square-300x267.jpg
NEC Labs America2024-10-21 00:00:002025-01-29 14:53:24iRAG: Advancing RAG for Videos with an Incremental ApproachTraffic cameras are essential in urban areas, playing a crucial role in intelligent transportation systems. Multiple cameras at intersections enhance law enforcement capabilities, traffic management, and pedestrian safety. However, efficiently managing and analyzing multi-camera feeds poses challenges due to the vast amount of data. Analyzing such huge video data requires advanced analytical tools. While Large Language Models (LLMs) like ChatGPT, equipped with retrieval-augmented generation (RAG) systems, excel in text-based tasks, integrating them into traffic video analysis demands converting video data into text using a Vision-Language Model (VLM), which is time-consuming and delays the timely utilization of traffic videos for generating insights and investigating incidents. To address these challenges, we propose TrafficLens, a tailored algorithm for multi-camera traffic intersections. TrafficLens employs a sequential approach, utilizing overlapping coverage areas of cameras. It iteratively applies VLMs with varying token limits, using previous outputs as prompts for subsequent cameras, enabling rapid generation of detailed textual descriptions while reducing processing time. Additionally, TrafficLens intelligently bypasses redundant VLM invocations through an object-level similarity detector. Experimental results with real-world datasets demonstrate that TrafficLens reduces video-to-text conversion time by up to 4× while maintaining information accuracy.
https://neclabs.wpengine.com/wp-content/uploads/2022/08/NEC-Labs-Blue-Logo-Square-300x267.jpg
0
0
NEC Labs America
https://neclabs.wpengine.com/wp-content/uploads/2022/08/NEC-Labs-Blue-Logo-Square-300x267.jpg
NEC Labs America2024-09-24 00:00:002025-03-28 10:22:07TrafficLens: Multi-Camera Traffic Video Analysis Using LLMsEnsuring high-quality video content for wireless users has become increasingly vital. Nevertheless, maintaining a consistent level of video quality faces challenges due to the fluctuating encoded bitrate, primarily caused by dynamic video content, especially in live streaming scenarios. Video compression is typically employed to eliminate unnecessary redundancies within and between video frames, thereby reducing the required bandwidth for video transmission. The encoded bitrate and the quality of the compressed video depend on encoder parameters, specifically, the quantization parameter (QP). Poor choices of encoder parameters can result in reduced bandwidth efficiency and high likelihood of non-conformance. Non-conformance refers to the violation of the peak signal-to-noise ratio (PSNR) constraint for an encoded video segment. To address these issues, a real-time deep learning-based H.264 controller is proposed. This controller dynamically estimates the optimal encoder parameters based on the content of a video chunk with minimal delay. The objective is to maintain video quality in terms of PSNR above a specified threshold while minimizing the average bitrate of the compressed video. Experimental results, conducted on both QCIF dataset and a diverse range of random videos from public datasets, validate the effectiveness of this approach. Notably, it achieves improvements of up to 2.5 times in average bandwidth usage compared to the state-of-the-art adaptive bitrate video streaming, with a negligible non-conformance probability below 10?2.
https://neclabs.wpengine.com/wp-content/uploads/2022/08/NEC-Labs-Blue-Logo-Square-300x267.jpg
0
0
NEC Labs America
https://neclabs.wpengine.com/wp-content/uploads/2022/08/NEC-Labs-Blue-Logo-Square-300x267.jpg
NEC Labs America2024-06-09 00:00:002024-09-05 13:05:13Deep Learning-Based Real-Time Quality Control of Standard Video Compression for Live StreamingAI/ML techniques have been used to solve systems problems, but their applicability to customize solutions on-the-fly has been limited. Traditionally, any customization required manually changing the AI/ML model or modifying the code, configuration parameters, application settings, etc. This incurs too much time and effort, and is very painful. In this paper, we propose a novel technique using Generative Artificial Intelligence (GenAI) technology, wherein instructions can be provided in natural language and actual code to handle any customization is automatically generated, integrated and applied on-the-fly. Such capability is extremely powerful since it makes customization of application settings or solution techniques super easy. Specifically, we propose ECO-LLM (LLM-based Edge Cloud Optimization), which leverages Large Language Models (LLM) to dynamically adjust placement of application tasks across edge and cloud computing tiers, in response to changes in application workload, such that insights are delivered quickly with low cost of operation (systems problem). Our experiments with real-world video analytics applications i.e. face recognition, human attributes detection and license plate recognition show that ECO-LLM is able to automatically generate code on-the-fly and adapt placement of application tasks across edge and cloud computing tiers. We note that the trigger workload (to switch between edge and cloud) for ECO-LLM is exactly the same as the baseline (manual) and actual placement performed by ECO-LLM is only slightly different i.e. on average (across 2 days) only 1.45% difference in human attributes detection and face recognition, and 1.11% difference in license plate recognition. Although we tackle this specific systems problem in this paper, our proposed GenAI-based technique is applicable to solve other systems problems too.
https://neclabs.wpengine.com/wp-content/uploads/2022/08/NEC-Labs-Blue-Logo-Square-300x267.jpg
0
0
NEC Labs America
https://neclabs.wpengine.com/wp-content/uploads/2022/08/NEC-Labs-Blue-Logo-Square-300x267.jpg
NEC Labs America2024-06-03 00:00:002024-09-05 12:25:19ECO-LLM: LLM-based Edge Cloud Optimization