Link to: Media Analytics
MEDIA ANALYTICS
PROJECTS
Link to: MA People
PEOPLE
Link to: MA Publications
PUBLICATIONS
Link to: MA Patents
PATENTS
Structure-and-Motion-Aware Rolling Shutter Correction
We make a theoretical contribution by proving that RS two-view geometry is degenerate in the case of pure translational camera motion. In view of the complex RS geometry, we then propose a convolutional neural network-based method which learns the underlying geometry (camera motion and scene structure) from just a single RS image and performs RS image correction. We propose a geometrically meaningful way to synthesize large-scale training data and identify a geometric ambiguity that arises for training.
Collaborators: Manmohan Chandraker
Learning Structure-And-Motion-Aware Rolling Shutter Correction Paper
1National University of Singapore 2NEC Labs America 3University of California, San Diego
IEEE Conference on Computer Vision and Pattern Recognition (CVPR), 2019 [Oral]
From a set of GS images and corresponding GS depth maps, we generate synthetic RS images with corresponding RS camera motions and RS depth maps for training our network. At testing, given a single real RS image, our network predicts an accurate RS camera motion and RS depth map, which are used for correcting RS effects in the input image.
Abstract
An exact method of correcting the rolling shutter (RS) effect requires recovering the underlying geometry, i.e. the scene structures and the camera motions between scanlines or between views. However, the multiple-view geometry for RS cameras is much more complicated than its global shutter (GS) counterpart, with various degeneracies. In this paper, we first make a theoretical contribution by showing that RS two-view geometry is degenerate in the case of pure translational camera motion. In view of the complex RS geometry, we then propose a Convolutional Neural Network (CNN)-based method which learns the underlying geometry (camera motion and scene structure) from just a single RS image and perform RS image correction. We call our method structure-and-motion-aware RS correction because it reasons about the concealed motions between the scanlines as well as the scene structure. Our method learns from a large-scale dataset synthesized in a geometrically meaningful way where the RS effect is generated in a manner consistent with the camera motion and scene structure. In extensive experiments, our method achieves superior performance compared to other state-of-the-art methods for single image RS correction and subsequent Structure from Motion (SfM) applications.
Image Resizing vs. Image Cropping
Performances of our networks when trained with cropped images or resized images and tested on image sets with: (a) wx-rotation only, (b) wy-rotation only, and (c) wz-rotation only.
Rectification Results on Synthetic RS Images
Qualitative comparisons on a synthetic RS image with a typical scene in KITTI Raw. The first row shows the input RS image, input RS image overlaid on ground truth GS image (pink and green colors indicate the intensity differences), ground truth undistortion flow, and ground truth depth map (bright and dark colors indicate small and large depth values respectively). The next three rows plot the results of our method (SMARSC), MH, and 2DCNN respectively with each row showing from left to right the rectified image, rectified image overlaid on ground truth GS image, estimated undistortion flow, and estimated depth map. Note that since MH and 2DCNN do not predict depths, we instead show the line detection result for MH and leave an empty figure for 2DCNN.
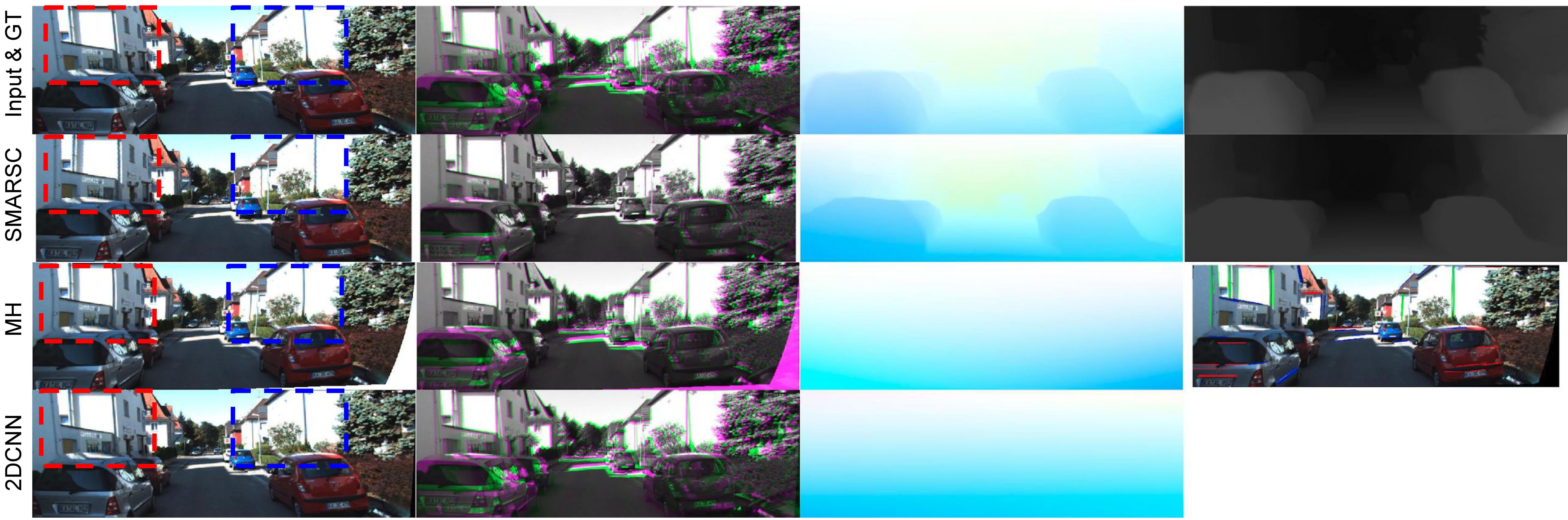
Quantitative comparisons on synthetic RS images with: (a) 6-DOF camera motion and (b) pure rotation.
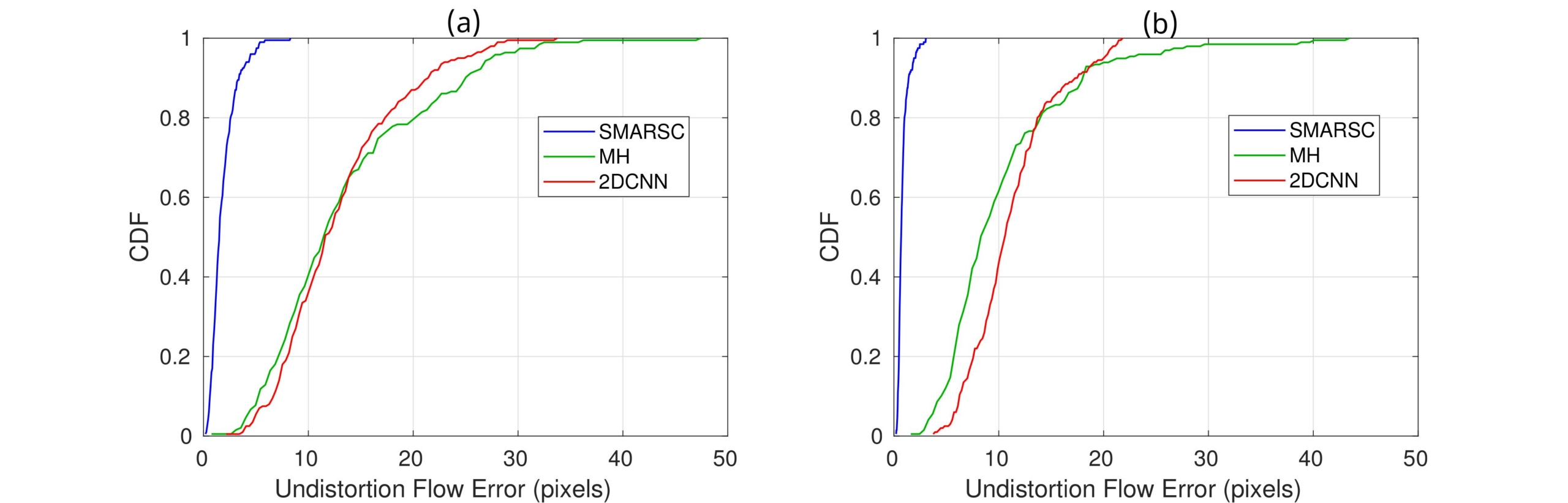
Rectification Results on Real RS Images
Qualitative comparisons on real RS images. The first column shows different input RS images, while the next three columns plot the results of our method (SMARSC), MH, and 2DCNN, respectively. Undistortion flow (and depth map) estimated by different methods for I1 in the above figure.
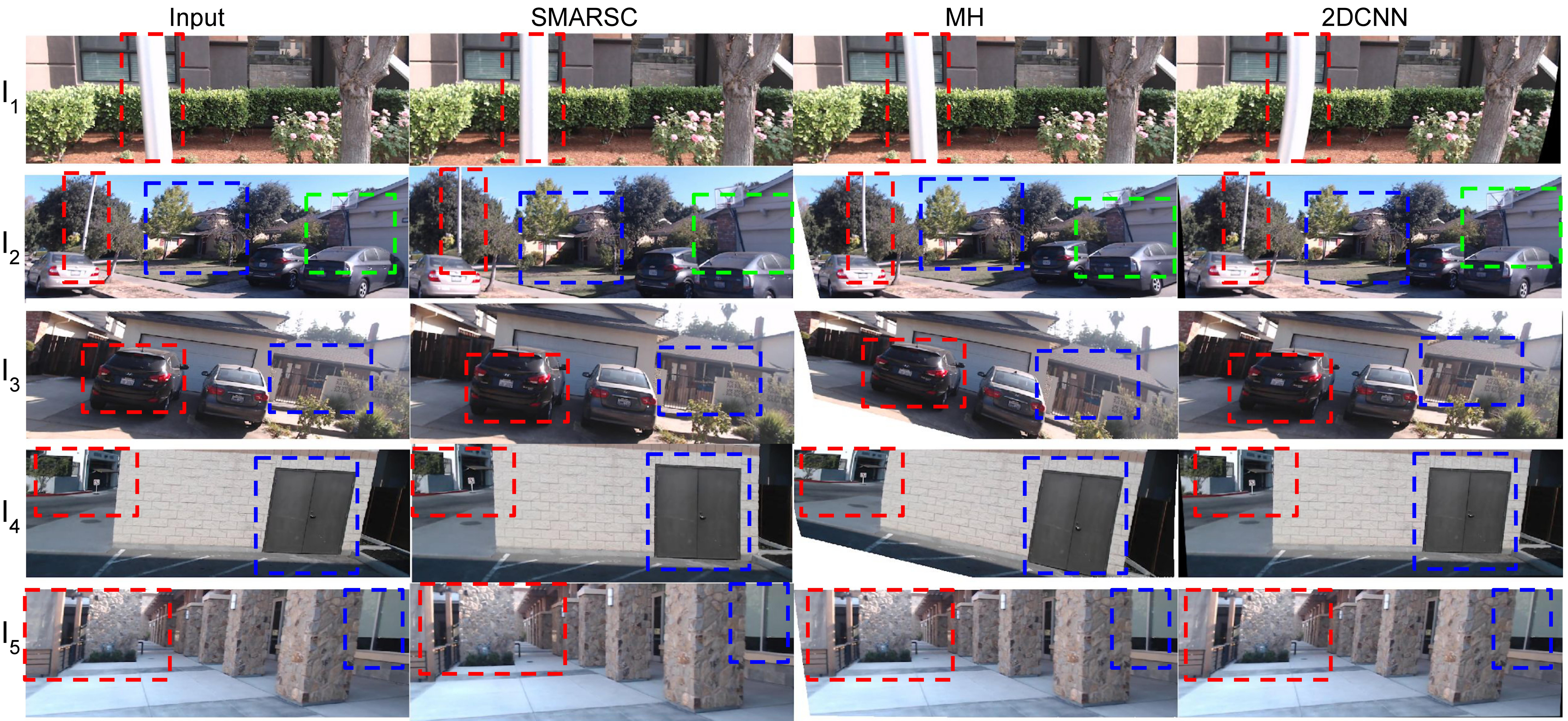
SfM Results on Real RS Images
Qualitative results of SfM with RS images in top view. Four equally-spacing pillars are indicated in the top image.
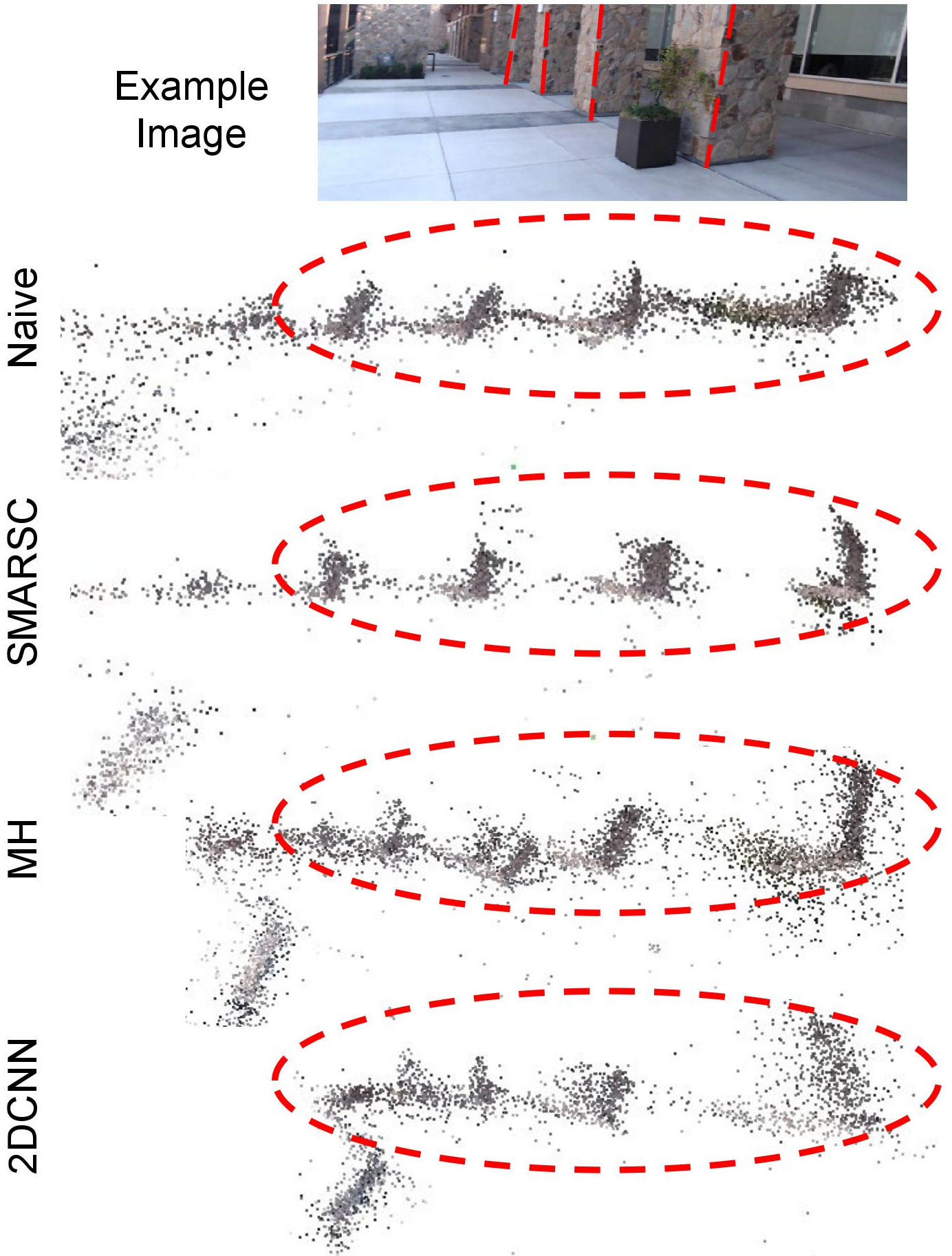
Acknowledgements
This work was done during Bingbing Zhuang’s internship at NEC Labs America. This work is also partially supported by the Singapore PSF grant 1521200082. This website template is inspired by this website.